
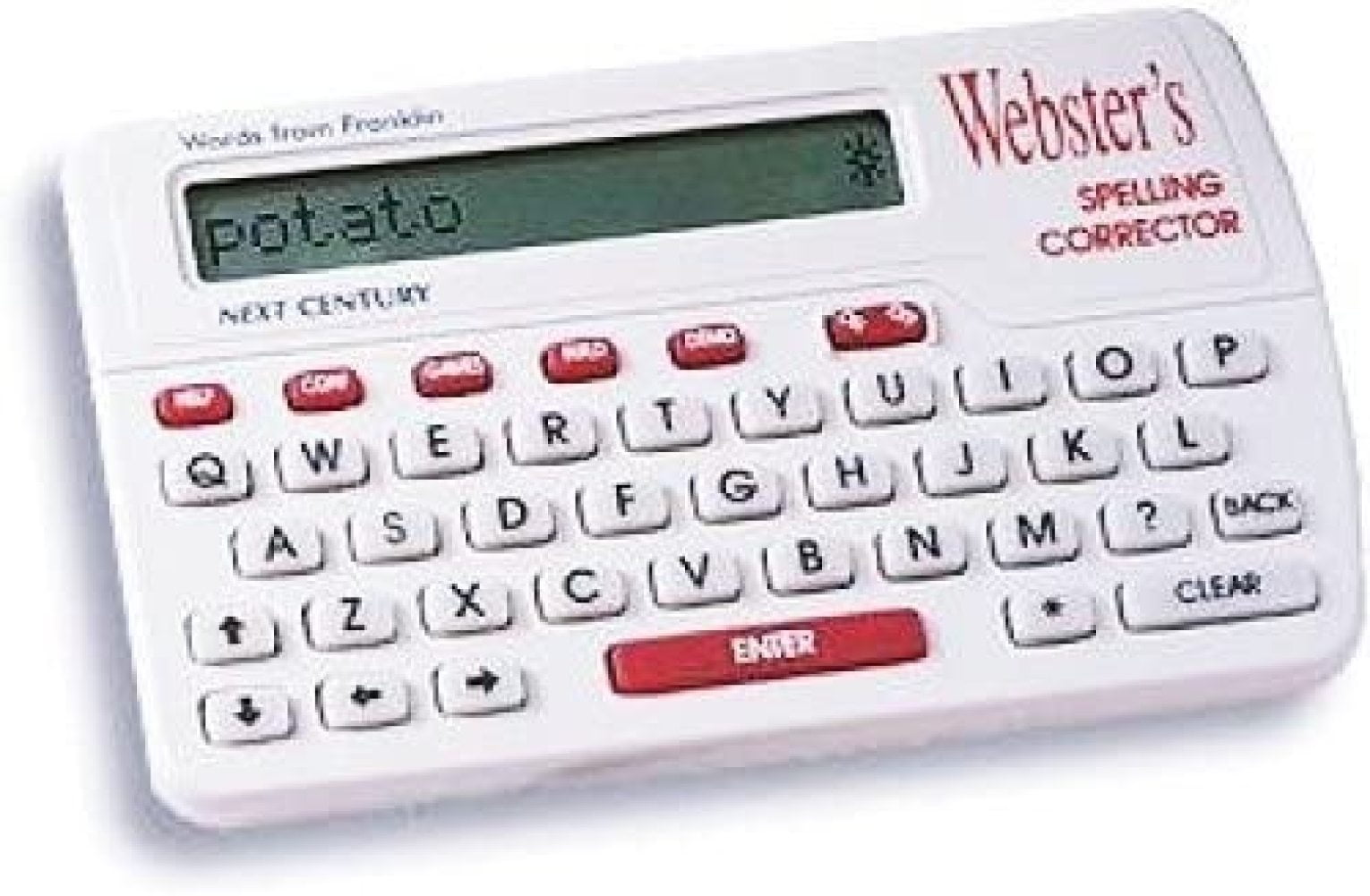
#WORD SPELLING CORRECTOR MANUAL#
However, manual annotation has limited scalability and is not conductive towards CPS assessments at scale. Modern research in CPS relies on theory‐driven measurements that are usually carried out as manual annotations over recorded logs of collaborative activities. Measurement of CPS skills has emerged as an essential aspect in educational assessment. These results give credence to the potential for automated approaches to help advance the assessment of CPS and to circumvent the time-intensive human annotation approaches that are typically used in these contexts.Ĭollaborative problem solving (CPS) is important for success in the 21st century, especially for teamwork and communication in technology‐enhanced environments.

Comparisons between such an automated approach and an approach based on human annotation to characterize student CPS behaviors revealed above average agreement. We further describe computational linguistic methods for automatically characterizing students’ display of various CPS skills in the task. In the current study, we describe the use of a simulation-based task on electronics concepts as an environment for higher education students to display evidence of their CPS competency. In a move to computer-based environments to support CPS assessment, innovative computational approaches are also needed to understand individuals’ CPS behaviors. Innovative approaches for assessing individuals’ CPS competency are necessary, as traditional assessment types such as multiple -choice items are not well suited for such a process-oriented competency. Implemented system that achieves high accuracy on this task.Ĭompetency in skills associated with collaborative problem solving (CPS) is critical for many contexts, including school, the workplace, and the military. International high-stakes English language assessments. Of proposed methods is demonstrated with an annotated corpus of 3,000 student essays from If the approximate topicĬan be known in advance, spelling correction can be biased towards the topic. The fourth approach looks at context beyond the text itself. In the thirdĪpproach, recognizing a misspelling as an instance of a recurring word can be useful for reranking. Local lexical neighborhood, using a very large Distributional Semantic Model. Second approach measures how well a candidate correction fits in the semantic fabric of the Local context is captured by word n-grams statistics from a Web-scale language model. The task is framed as contextually-informed re-ranking of correction candidates. This paper presents an investigation on using four types of contextual informationįor improving the accuracy of automatic correction of single-token non-word misspellings.
